We will have a breakthrough for hydrology research internationally
Key to the success of Forest Flows is developing a wireless datalogger network that can collect sensor data from multiple locations in a catchment and transmit it back to the office. However, planted forests are a very difficult environment to operate in as electronics are damaged by the damp environment, locations are remote, and signal is reduced by the terrain and vegetation. Existing expensive datalogger technology does not work well in forests.
Scion collaborated with the New Zealand technology Research and Design company INFACT Ltd, who successfully developed a fit-for-purpose, low-cost dataloggers able to meet the above challenges. The FlowLab dataloggers were built by New Zealand company Quick Circuit Ltd, and can operate five different types of sensors. This is a great example of NZ Inc. that successfully developed a world-leading technology.
Wireless mesh network
The wireless datalogger network will be installed at each primary and secondary site to collect real time and concurrent measurements of precipitation, throughfall, transpiration, diurnal tree growth, canopy evaporation, soil moisture and water potential, stream flow, groundwater, and stream water nitrate.
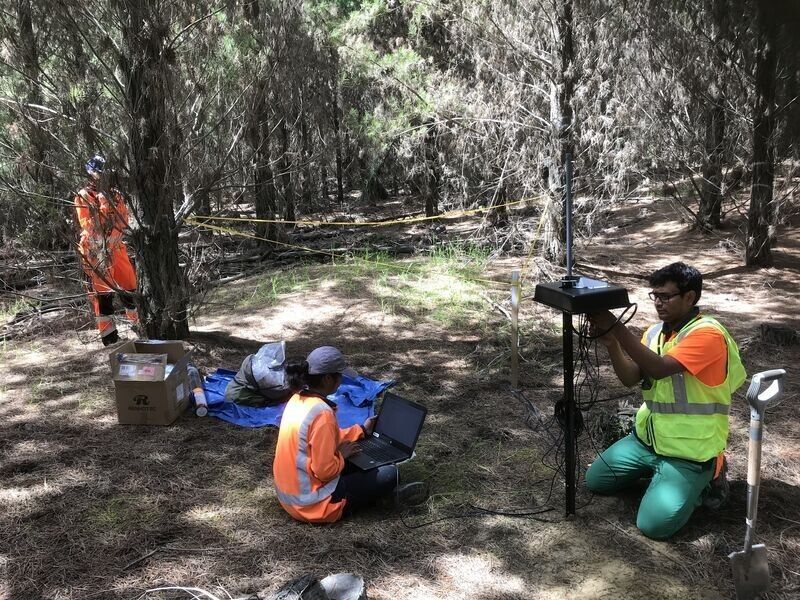
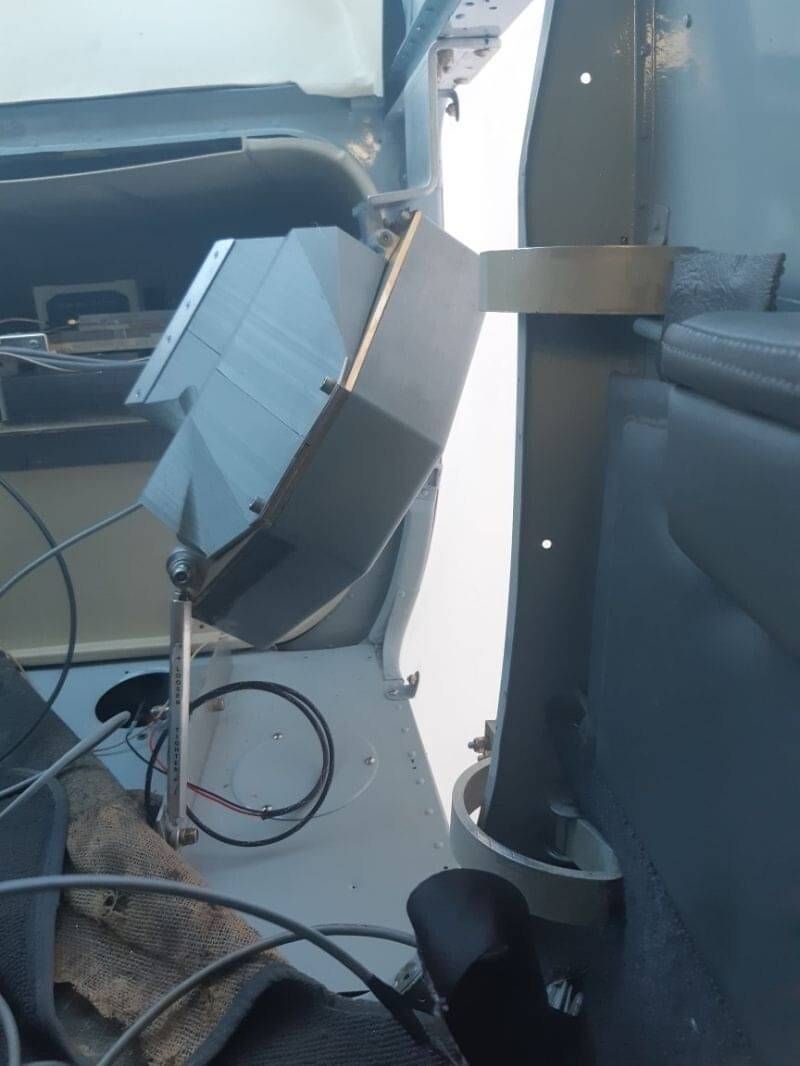
Measuring soil moisture from the air
The jewel of the Forest Flows crown is SlimSAR L- and P-Band synthetic aperture radar (SAR). This new radar technology from the USA can measure soil moisture from a plane to a depth of 1.5m. If successful, SlimSAR will provide a faster way to map soil properties and soil moisture across forested catchments and provide new insights on the mysteries of water retention and release in planted forests. Once we have a licence to operate, we will start collecting data this year.
Digital Twin - Pulse of the forest
The bold goal of this programme is to create a new biophysical forest hydrology model combining cutting edge remote sensing techniques with terrestrial based measurements, integrating data and enabling scale, from tree, to stand, to forest to catchment, as a digital model applicable to planted forests all over New Zealand.
These extrapolations will be made possible through detailed analysis and simulation capability, and coupled with powerful tools including visualisation and enabling access to insights, achieving this Forest Digital Twin will be a major breakthrough for hydrology research internationally.
The Forest Digital Twin as a capability
Connect and collect
Remote-sensing measurements, including L- and P-Band radar, hyperspectral imaging and LiDAR will be linked to terrestrial measurements at six monitoring sites in forested catchments across New Zealand.
NIWA has deployed sensors to measure the climate, groundwater, streamwater, and other measurements with their Lora sensor network. Together data collected by Scion and NIWA sensors will provide a real time understanding of water use, water retention and water release in catchments. Forest Flows has in total 19 different types of sensors and collectors at each primary site.
In addition, periodic terrestrial measurements will provide catchment spatial data including tree species biomass productivity, tree species leaf area index, soil physical properties mapping, bedrock and ground water mapping, precipitation soil residence time, soil and groundwater nitrate fluxes, and seasonal water sources for trees.
Integrate
To manage the 300,000+ daily observations, a big data pipeline was developed from scratch. A New Zealand first, Scion has developed the Forest Flows Big Data Kafka Pipeline that can seamlessly stream clean, summarise, and store big data arriving in real-time from the forest. The cloud storage of terrestrial data will make it easier for national and international collaborators to access and use the data, as well as facilitate collaboration.
Kafka will enable the fusion of intensive terrestrial site monitoring with remote sensing.
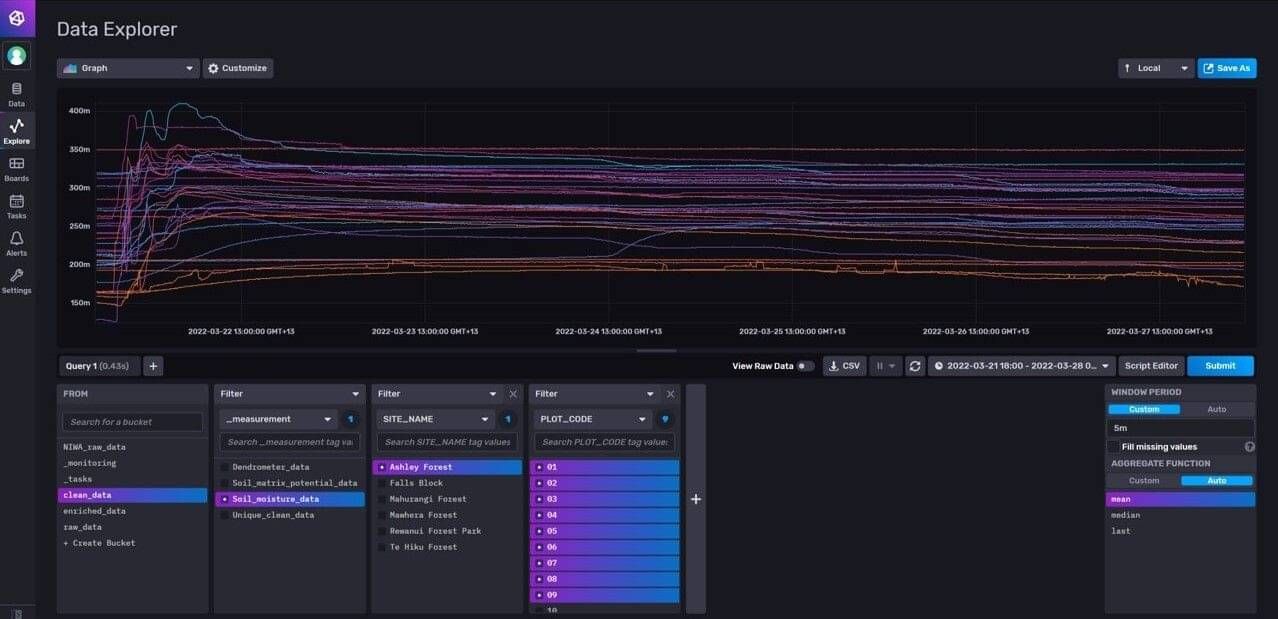
InfluxDB provides an easy interface with the Kafka database to query and extract large datasets from multiple sensors and sites in seconds.
Analyze and simulate
Success will require innovative analysis and processing methods to link the fine-scale terrestrial measurements to the remote sensing data to create robust relationships for extrapolation, enabling the characterization of planted-forest hydrology across different tree species, catchment positions, soil/geology, climates and seasons.
The bio-physical model will predict forest hydrological fluxes across a range of New Zealand planted forests by upscaling linked temporal and spatial data.
Insights and visualisation
The output will enable fast, cost-effective collection of quality data from any area – including remote forests – that can then be quickly translated into accurate and defensible predictions of hydrology fluxes.
Providing the required information to optimise water use and water quality in planted forests.
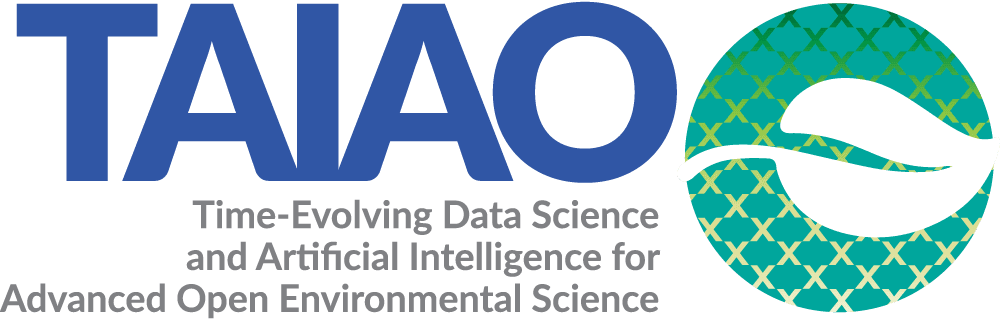
Machine learning and AI
Forest Flows has developed a key collaboration with the MBIE Time-Evolving Data Science/Artificial Intelligence for Advanced Open Environmental Science (TAIAO) data science programme led by the University of Waikato’s Artificial Intelligence Institute. This collaboration provides valuable synergies to both programmes.
Forest Flows will provide real time environmental data so that the Artificial Intelligence Institute can develop its TAIAO platform machine learning (ML)/artificial intelligence prototype. In conjunction with this, Forest Flows will develop a prototype cloud-based database frame that can house real time environmental big data for Forest Flows and the TAIAO platform.
The TAIAO programme will develop ML tools to analyse Forest Flows real time big data that would be challenging to analyse with traditional statistical methods.